GLIMMERHMM DOWNLOAD
Open in a separate window. They are also re-configurable and include several types of probabilistic submodels which can be independently combined, such as Maximal Dependence Decomposition trees and interpolated Markov models. National Center for Biotechnology Information , U. The evidence parameter E is defined by feature vectors, which record each evidence source's predictions at each nucleotide in the sequence. Note that the tuning of these extra parameters was performed on the first of two test sets; to avoid undesirable post hoc effects as a result of 'peeking' at the test set, our final results were measured on a second, unseen, test set described below. The effects of specific feature states on the modeling of human This step is optional to the user but many times a manual tuning of the system could improve the accuracy of the predictions.
Uploader: | Dotaxe |
Date Added: | 9 December 2013 |
File Size: | 15.98 Mb |
Operating Systems: | Windows NT/2000/XP/2003/2003/7/8/10 MacOS 10/X |
Downloads: | 5054 |
Price: | Free* [*Free Regsitration Required] |
JIGSAW, GeneZilla, and GlimmerHMM: puzzling out the features of human genes in the ENCODE regions
Although we are often asked this question by prospective users gkimmerhmm our gene finders, we know of very few studies addressing this most practical issue. The third difference was in the use of RefSeq genes. A post-processing phase allows us to impose a minimum isochore size by identifying predicted isochores smaller than the minimum allowable size and progressively combining them with their neighbors until all remaining isochore segments satisfy size constraints and such that no two isochores of the same class are adjacent.
Parameters to the program include: As any species-specific gene finder, GlimmerHMM needs a training data set containing as many glim,erhmm possible complete coding sequences from the organism genome for which the gene prediction is intended.
In the training procedure of GlimmerHMM the threshold for calling a sequence a real splice site is chosen by examining tlimmerhmm trade-off in the false positive rate. Position k marks an index in S.

The additional parameters were R 3'a multiplicative factor that adjusts the existing L 3' mean 3' UTR length parameter; and O poly 'poly-A optimism'another multiplicative factor that is applied to the pre-logarithm WAM score. The system creates a sorted list of thresholds, adjusting the scoring function so that it will miss 1,2,3, etc. To evaluate the utility of various evidence tracks in JIGSAW, we performed a series gliimmerhmm experiments in which individual tracks were progressively added to the gene finder's set of available inputs.
P amino c M sp was estimated by observing frequencies of amino acids in the set of training signal peptides; P c amino c was estimated by observing the codon usage statistics of the training genes.
Sign In or Create an Account.
JIGSAW, GeneZilla, and GlimmerHMM: puzzling out the features of human genes in the ENCODE regions
The trend appears to be effectively flat for sample sizes above 6, genes data not shown. These genes would form a sound training data set if their number would be large enough. This is also valid for GlimmerHMM. Because the WAM was trained via simple maximum likelihood and is, therefore, not guaranteed to provide optimal discrimination power for the gene finder as a whole [ 3 ], we incorporated two additional parameters related to this state and explored a broad range of values for these parameters in an attempt to discover a maximally discriminative parameterization.
Evaluation of training data quantity As a final experiment, we addressed the perennial question of how much training data would be sufficient to achieve near-optimal performance for an ab initio gene finder. An additional complication arises out of the use of different training protocols, which can have a profound effect on the performance of a single system [ 3 ], making interpretation of the differences between systems, absent knowledge of precisely how they were trained, very risky indeed.
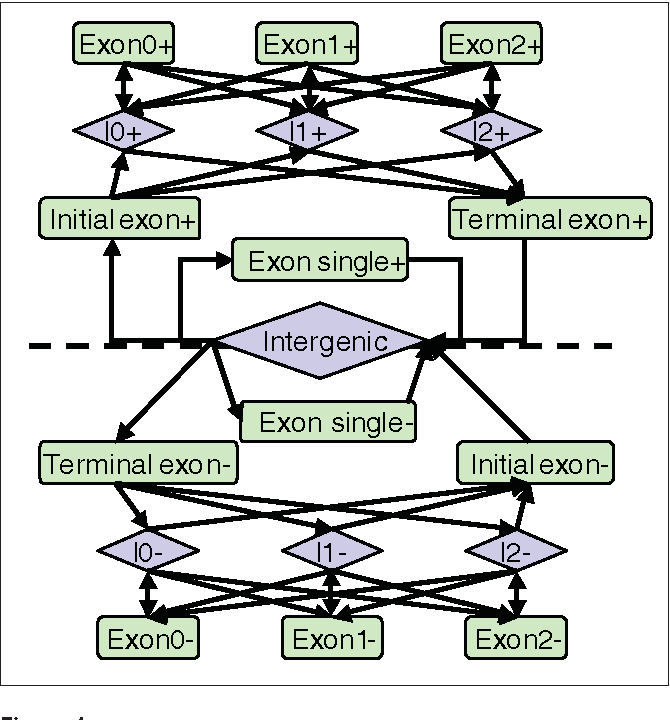
Please review our privacy policy. This is an open access article distributed under the terms of the Creative Commons Attribution License http: Basic local alignment search tool. For the default case, when no isochores are considered, there will be only one line with the foillowing info: The dashed line in the middle separates the positive strand and negative strand portions of the model.
We would also point out that sequencing centers have now completed draft genomes for hundreds of additional species, with many more to come. To download GlimmerHMM please click here.
As a final experiment, we addressed the perennial question of how much training data would be sufficient to achieve near-optimal performance for an ab initio gene finder. The Arabidopsis Genome Initiative, "Analysis of the genome sequence of the flowering plant Arabidopsis thaliana", Nature.
The decision to explicitly model only the 5' end of a CpG island in the GHMM was based on our observation that predicted CpG islands often overlapped the 5' region of a coding sequence CDS; data not shown. Though a number of promising approaches have been investigated, an ideal suite of tools has yet to emerge that can provide near perfect levels of sensitivity and specificity at the level of whole genes.
Putative signal peptide sequences S were evaluated by the signal peptide model M sp via:. Two consensus sequences were allowed for this signal: If not specified, the training directory is by default the current working directory. As an incremental step in this direction, it is hoped that controlled gene finding experiments in the ENCODE regions will provide a more accurate view of the relative benefits of different strategies for modeling and predicting gene structures.
Accurate individual evidence sources were identified as well as evidence combinations, where accuracy was dependant on the presence of multiple tracks of evidence.
BioinformaticsVolume 20, Issue 16, 1 NovemberPages —, https: Predicting complete protein-coding genes in human DNA remains a significant challenge. Although the gene finder conforms to the overall mathematical framework of a GHMM, additionally it incorporates splice site models adapted from the GeneSplicer program and a decision tree adapted from GlimmerM.
Comments
Post a Comment